Data Aggregation in Financial Services Explained
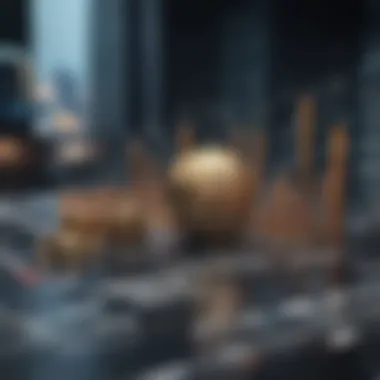
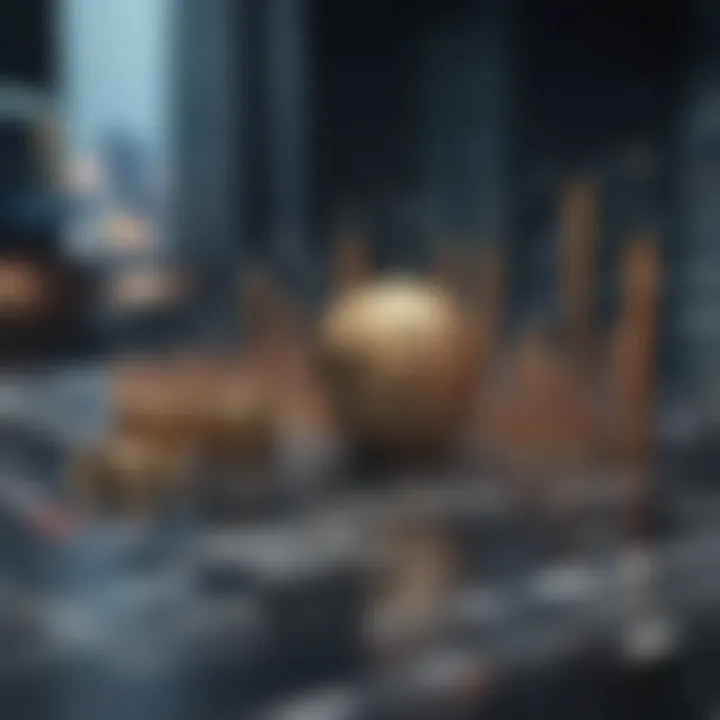
Intro
Data aggregation in financial services represents a crucial paradigm that significantly impacts investment strategies and decision-making processes. As financial markets become increasingly complex and data-driven, understanding the mechanisms of data aggregation is essential for both novices and seasoned investors. This understanding enables informed choices and enhances the potential for profitable returns.
This article will dissect the concept of data aggregation, elucidating its role within the financial sector. Various methods of data collection, integration, and analysis will be explored. Further, the influence of technology and regulatory considerations will be examined, along with anticipated future trends in data aggregation.
Key Financial Terms
Definitions
The following terms are fundamental to grasp the concept of data aggregation in the finance realm:
- Data Aggregation: The process of compiling data from various sources to form a comprehensive database. It enables the analysis of large datasets, identifying patterns and trends.
- Investment Strategies: Systematic approaches to investing, designed to maximize returns while managing risk. These strategies often rely on aggregated data to assess market conditions and forecast performance.
- Decision-Making Processes: Structured activities that involve analyzing data to make informed choices in financial contexts.
Examples of Use
- A investment firm might use data aggregation to collect stock prices and trading volume from different exchanges to develop a comprehensive view of market trends.
- Financial analysts often rely on aggregated economic indicators such as GDP growth, unemployment rates, and consumer spending data to inform their assessments.
Expert Tips
Beginner Strategies
For new investors, understanding data aggregation can be overwhelming. However, a few strategies can mitigate this:
- Start Small: Focus on a manageable subset of data, such as specific sectors or major indices.
- Utilize Tools: Leverage platforms that offer aggregated data reports. Many financial websites provide insights, analysis, and integrated data that can ease the learning process.
Advanced Techniques
Experienced investors can take advantage of more complicated aggregation techniques:
- Custom Analytics: Creating tailored analytics dashboards that synthesize data from various channels can yield deeper insights.
- Machine Learning: Implementing machine learning algorithms can help analyze vast amounts of aggregated data quickly, uncovering patterns that would be hard to identify manually.
"In today's financial landscape, the effective use of aggregated data can mean the difference between success and failure in investment management."
This comprehensive overview of data aggregation in financial services aims to equip investors with vital knowledge and strategies for navigating this dynamic field. By embracing the insights delivered through data aggregation, investors can enhance their decision-making capabilities and, ultimately, their financial outcomes.
Intro to Data Aggregation in Financial Services
Data aggregation serves as a crucial pillar within the financial services sector, enabling organizations to compile and analyze vast amounts of data from various sources. This practice has a significant impact on enhancing decision-making and developing more effective investment strategies. In an industry where information translates to power, understanding data aggregation equips both novice and seasoned investors with the tools necessary to navigate complex financial landscapes.
Definition of Data Aggregation
Data aggregation refers to the process of collecting and organizing data from multiple sources into a central repository. This collection can include market data, customer information, and transactional records. The essence of data aggregation in financial services lies in its ability to streamline and standardize information, making it more accessible for analysis. Businesses leverage data aggregation to gain insights that drive strategic decisions, improve operational efficiencies, and ultimately enhance customer experiences.
This process often involves utilizing various techniques and technologies that ensure the quality and relevance of the data collected. Users need to differentiate between raw data and processed data. Raw data requires careful handling, while processed data offers actionable insights.
Additionally, data aggregation enables organizations to identify trends and patterns that might otherwise go unnoticed. For instance, an investment firm can aggregate data on market conditions, competitor performance, and economic indicators to formulate sound investment strategies.
Historical Context
The concept of data aggregation in financial services is not new; it has evolved significantly over the years. Historically, financial institutions relied on manual methods of data collection, which were often time-consuming and prone to errors. In the early days, data was gathered through routine reports, physical records, and direct client interactions. However, the rise of digital technology brought about a paradigm shift.
With the advent of the internet in the 1990s, financial services saw the beginning of automated data collection methods. This era marked the transition from paper-based systems to digital databases. It allowed firms to capture data at a much larger scale and at a quicker pace than ever before.
In the 2000s, the introduction of APIs and web scraping solidified data aggregation techniques, allowing financial firms to access third-party data seamlessly. The ongoing advancements in cloud computing and machine learning applications further revolutionized the efficiency of data aggregation processes.
Today, financial service providers recognize that data aggregation is essential for compliance, risk management, and customer relationship management. This historical perspective underscores the evolutionary journey of data aggregation and its pivotal role in shaping modern financial services.
Importance of Data Aggregation
Data aggregation plays a crucial role in the financial sector. It allows organizations to collect, organize, and analyze vast amounts of data from various sources. This process helps them make informed decisions that can enhance efficiency and profitability. By leveraging data aggregation, businesses can gain deeper insights into trends and market dynamics, resulting in better strategic outcomes.
Enhancing Decision-Making
The informed decision-making process is central to successful financial management. Data aggregation provides access to comprehensive datasets. This access enables financial institutions and investors to analyze patterns and relationships that may not be visible when data is isolated. For example, when examining market conditions, integrating market data with customer data allows institutions to forecast trends more accurately. This ability is not just beneficial but necessary in today’s fast-paced financial environment.
Moreover, data aggregation can help mitigate risk. By consolidating transactional data, organizations can identify anomalies and potential fraud much sooner. The early detection of these issues improves overall security and customer trust. Ultimately, the aggregation of diverse data sources empowers decision-makers to consider multiple scenarios before taking action.
Improving Investment Strategies
Investment strategies are often grounded in data analysis. Data aggregation enhances the robustness of these strategies. With comprehensive data, investors can assess the performance of various assets more effectively. By analyzing past trends and present market conditions through aggregated data, investors can forecast future performance with a higher degree of certainty.
In addition, many financial firms adopt advanced analytics tools. These tools can distill complex datasets into actionable insights. For example, by using machine learning models on aggregated data, firms can optimize their portfolios and enhance return potential. This trend is reshaping how investment strategies are designed. As such, an organization's long-term success relies heavily on its capacity to aggregate and analyze data effectively.
Types of Data Used in Financial Services
Data aggregation relies on various types of data that can be analyzed to drive insights and inform decisions in the financial sector. Understanding these types is crucial. It shapes how businesses interact with their clients and how they implement their strategic initiatives. Below are key categories of data relevant to financial services:
Market Data
Market data refers to information pertaining to the financial markets, including price movements, trading volumes, and historical performance of assets. This type of data is essential for several reasons:
- Timely Insights: Access to real-time market data allows financial professionals to make informed decisions swiftly, reacting to changes in the market.
- Risk Management: Understanding market trends helps in identifying potential risks and opportunities, thus enhancing risk management practices.
- Investment Strategy Development: Investors depend on market data to assess entry and exit points, ensuring a robust approach to portfolio management.
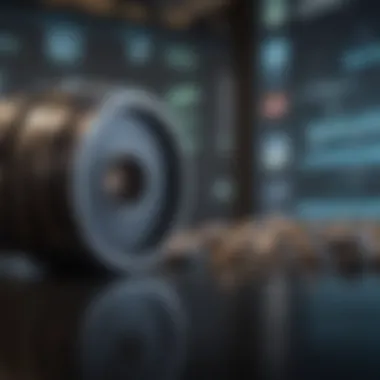
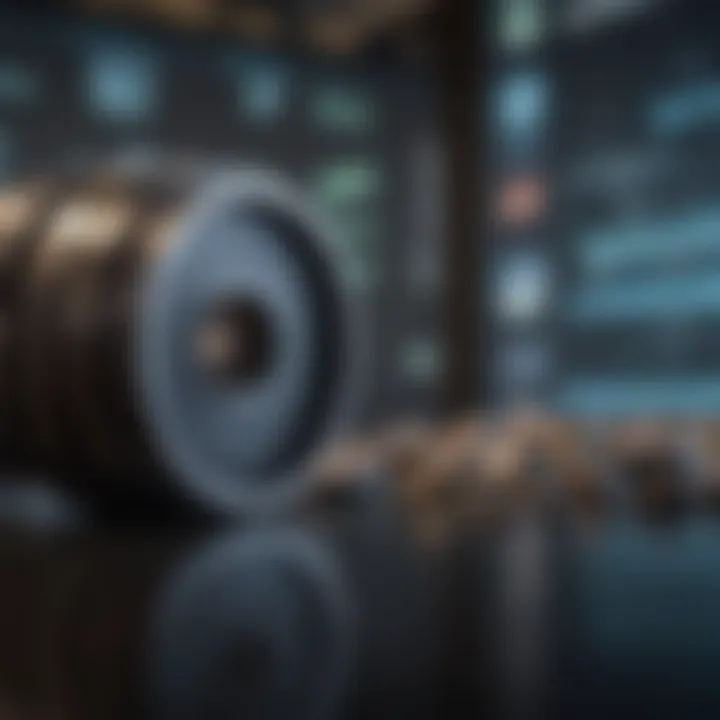
Market data can be obtained from various sources including exchanges and financial news networks. It is vital for staying competitive in today’s fast-paced financial environment.
Customer Data
Customer data is a wealth of information that sheds light on client behavior, preferences, and demographics. This data serves several purposes:
- Personalization: By analyzing customer data, financial institutions can tailor their services to meet individual needs, promoting enhanced customer satisfaction.
- Customer Segmentation: Financial services can divide their clients into distinct groups, allowing them to allocate resources more efficiently and target marketing efforts accurately.
- Predictive Analytics: Utilizing past customer behaviors can help predict future actions, aiding businesses in proactive decision-making.
Banks and financial advisors often collect this data through surveys, transaction records, and interactions with clients. Understanding customer data is paramount for fostering loyalty and retaining clients in a competitive market.
Transactional Data
Transactional data is generated through each financial transaction a customer makes, including deposits, withdrawals, and purchases. This category has distinct advantages:
- Operational Efficiency: Aggregating transactional data can reveal patterns that inform operational changes, reducing costs and enhancing service delivery.
- Fraud Detection: Monitoring transaction data helps identify unusual patterns, which is crucial for fraud prevention and risk management.
- Comprehensive Reporting: Firms can generate detailed financial reports using aggregated transactional data, offering insights for regulatory compliance and strategic planning.
This data is intrinsically connected with customer data, providing a clearer picture of client activity over time.
"The ability to aggregate and analyze various types of data is transformative for the financial sector. It allows firms to stay ahead and foster deeper client relationships."
Methods of Data Aggregation
Data aggregation serves as a cornerstone in the financial services sector, fundamentally shaping how data is collected, processed, and utilized. Understanding the methods of data aggregation is crucial as these techniques directly impact the quality of insights derived from aggregated data. By applying appropriate methods, institutions can enhance their operational efficiency and achieve strategic goals. In this section, we will explore three pivotal methods: API integration, web scraping techniques, and manual data collection.
API Integration
API integration is a streamlined method for data aggregation, allowing systems to communicate effectively. APIs, or Application Programming Interfaces, enable the seamless transfer of data between different software applications. This integration offers numerous benefits, such as real-time data access and automated data updates.
Financial organizations leverage API integration to connect with external data sources like market exchanges or third-party vendors. This approach allows firms to obtain essential market data without engaging in cumbersome manual input.
Moreover, APIs come with built-in security features that protect sensitive financial data, addressing privacy concerns in an increasingly regulated environment. Adopting API integration not only enhances data accuracy but also empowers financial analysts to make timely decisions based on live data.
Web Scraping Techniques
Web scraping techniques involve extracting data from websites using automated tools or scripts. In the financial services arena, web scraping is particularly valuable for gathering market trends, competitor insights, and consumer feedback. By systematically collecting data from various online sources, organizations can gain a comprehensive view of market dynamics.
The primary advantage of web scraping lies in its ability to collect vast amounts of data quickly. This method eliminates the need for manual searches across multiple websites, saving both time and resources. However, practitioners must remain aware of the legal and ethical considerations surrounding data scraping. Respecting a website’s terms of service and ensuring compliance with data protection regulations is essential for sustainable operations.
Manual Data Collection
Manual data collection involves the traditional approach of gathering data through surveys, interviews, or direct observation. While this method may seem outdated, it retains relevance, especially for unique cases where automated methods might not suffice. Financial services often require qualitative insights that can only come from human interaction, making manual collection indispensable.
This method does come with challenges, such as the potential for human error and time consumption. However, when executed effectively, manual data collection can yield rich insights not captured by automated tools. For instance, in-depth interviews with investment clients might reveal underlying motivations that statistical data alone cannot convey.
"Each method of data aggregation has its strengths and weaknesses, and understanding these elements helps financial entities choose the appropriate approach for their specific needs."
In summary, the methods of data aggregation—API integration, web scraping techniques, and manual data collection—each present distinct advantages for financial services. By thoughtfully selecting and implementing these methods, financial firms can optimize data utilization for enhanced decision-making and strategic planning.
Technological Innovations Driving Data Aggregation
Data aggregation in financial services relies heavily on technological innovations. These innovations enhance data collection, processing, and analysis capabilities. Understanding this relationship is vital for investors and financial professionals who want to leverage data effectively.
Machine Learning Applications
Machine learning is a powerful tool in data aggregation. It enables systems to learn from data patterns and improve their predictions over time. In finance, machine learning algorithms can analyze vast datasets to identify trends and anomalies.
Key benefits of using machine learning include:
- Improved Accuracy: Algorithms learn from historical data, leading to more accurate predictions.
- Automation: Time-consuming tasks can be automated, increasing efficiency.
- Risk Management: Machine learning helps in risk assessment by identifying high-risk transactions or areas.
Investors can benefit from these applications by using platforms that incorporate machine learning. For instance, firms like Betterment and Wealthfront utilize such technologies to optimize investment strategies.
Cloud Computing Solutions
Cloud computing has transformed the way financial data is stored and accessed. It offers scalable solutions that can handle large volumes of data without significant capital expenditure. The advantages of cloud computing in data aggregation include:
- Scalability: Organizations can quickly scale up or down based on data needs.
- Cost-Effectiveness: Reduced need for physical infrastructure lowers costs for companies.
- Collaboration: Teams can access data from various locations, facilitating better collaboration.
One example of cloud computing in finance is Salesforce, which provides robust data integration tools. With such solutions, financial institutions can aggregate data from multiple sources into a unified platform more easily than ever before.
Challenges in Data Aggregation
Data aggregation in financial services presents several challenges that can hinder its effectiveness. Understanding these challenges is essential for investors and financial institutions to mitigate risks and enhance the benefits of data processing. Addressing these challenges is crucial for the reliability of the aggregated information. Two primary issues arise in this context: data privacy concerns and data quality issues.
Data Privacy Concerns
Data privacy is a significant challenge in the process of data aggregation. Financial institutions handle sensitive client information, including personal identification details and transaction histories. Breaches of this data can lead to severe consequences, including financial loss and reputational damage.
- Regulatory Compliance: Institutions must comply with various regulations such as the General Data Protection Regulation (GDPR) and the California Consumer Privacy Act (CCPA). These regulations outline rules regarding the collection, usage, and protection of personal data. Non-compliance can result in hefty fines and legal repercussions.
- Consumer Trust: Maintaining customer trust is vital. Any perceived breach of privacy can result in clients withdrawing their business. Safeguarding data is not just a legal requirement; it is fundamental to building long-term relationships with clients. Institutions must invest in secure data systems and transparent policies to alleviate concerns.
- Data Minimization: Only collecting the data necessary for specific purposes can help reduce risks. This practice involves assessing what data is essential for aggregation and cutting down on extraneous information.
In summary, managing data privacy effectively is crucial for financial institutions to protect both themselves and their clients.
Data Quality Issues
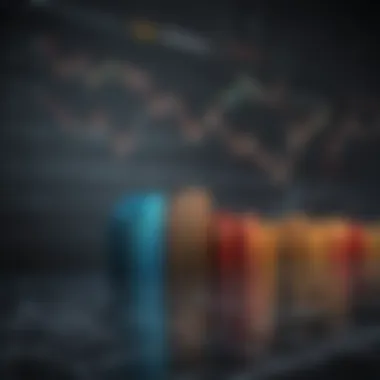
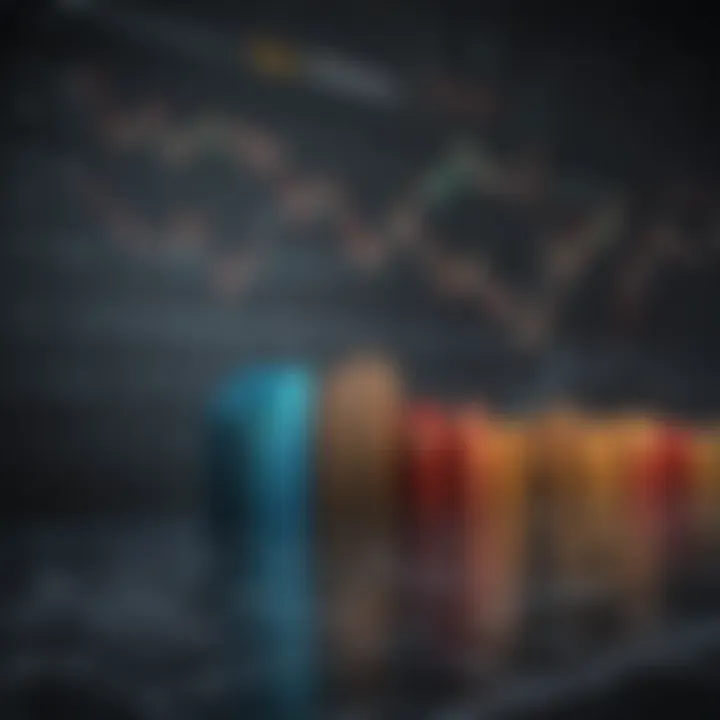
Data quality is another prominent challenge in data aggregation. Poor quality data can lead to erroneous insights, potentially harming investment strategies and decision-making processes.
- Accuracy: Ensuring data accuracy is essential. Data must be free of errors and represent true values. Inaccurate data can lead to misguided strategic decisions. Establishing rigorous data entry practices and regular audits can mitigate this risk.
- Completeness: Incomplete data can skew analysis. Missing information on transactions or customer profiles can lead to overlooking significant patterns or insights. Organizations must strive to gather complete data sets to enhance analytical reliability.
- Timeliness: Data must be aggregated in real-time to be relevant. Slow updating processes can result in outdated information, which is particularly detrimental in fast-paced financial markets. Institutions should implement efficient data aggregation processes to ensure data freshness.
- Consistency: Inconsistencies can arise from data collected from multiple sources. Different formats or standards can complicate the aggregation process. Creating standardized protocols for data collection can aid in achieving uniformity across datasets.
"High-quality data is essential for making informed decisions in the financial sector."
Understanding and effectively managing these challenges can strengthen the role of data aggregation in improving financial services.
Regulatory Framework for Data Aggregation
The regulatory framework governing data aggregation in financial services holds great importance. It is essential for ensuring the integrity, security, and ethical use of data. Regulations create a structure that companies must follow, which helps to protect consumers. Investors must be aware of these frameworks, as they can impact the availability and usage of data used in various financial practices.
Compliance Requirements
Compliance with regulations is a priority for institutions involved in financial services. Strict guidelines dictate how data can be collected, stored, and processed. These requirements arise from various laws and standards, such as data protection regulations and financial industry-specific rules.
- Data Privacy Laws: Laws require firms to obtain consent from customers before collecting personal data. This ensures individuals understand how their data will be used.
- Financial Reporting Standards: Financial institutions must adhere to specific standards when presenting data to ensure transparency.
- Audit Trails: Keeping clear records of data usage is crucial. This enables companies to demonstrate compliance and address any regulatory inquiries efficiently.
Failure to meet these requirements can lead to severe penalties. Institutions may face hefty fines, and their reputational damage can deter clients. Therefore, being compliant is not just about following rules; it is also about maintaining trust with consumers.
Impact of Regulations on Practices
The regulations influence how companies operate, shaping their data aggregation methods and strategies. Companies often adapt their systems to comply with evolving regulations. This can incite various changes in practices:
- Investment in Technology: Financial services must invest in scalable technologies that can manage compliance seamlessly. For example, automated compliance systems help track changes in data usage and regulations.
- Data Minimization: Regulations often require firms to limit data collection to what is essential for their operations. This means companies must assess what data is truly necessary for their functions.
- Training and Awareness: Ensuring that employees understand compliance practices is vital. Regular training sessions can foster a clear understanding of ongoing regulatory requirements.
Adapting to a complex regulatory environment can be challenging. However, it can also lead to more refined data strategies that improve data quality and security.
Future Trends in Data Aggregation
The future of data aggregation in financial services holds significant promise. As the landscape continues to evolve, understanding these trends is essential. The integration of advanced technologies, regulation updates, and changing consumer behavior all play pivotal roles in shaping this domain. These trends not only enhance the efficiency of data processing but also improve the accuracy of insights derived from data.
Integration of AI and Data Analytics
The integration of Artificial Intelligence (AI) and data analytics is set to transform data aggregation significantly. AI can analyze vast amounts of data at an unprecedented speed and accuracy. This capability enables financial institutions to refine their decision-making processes. For instance, AI algorithms can detect patterns that humans may overlook, providing deeper insights into consumer behavior and market trends.
Benefits of AI Integration
- Automation: Automating data aggregation tasks can reduce human error and increase efficiency.
- Predictive Analytics: AI can use historical data to forecast market movements, aiding in better investment decisions.
- Risk Management: Enhanced risk assessment through real-time data analysis helps institutions mitigate potential losses.
Organizations that leverage AI-driven data aggregation tools gain a competitive edge. They can respond more quickly to market changes and meet consumer demands efficiently.
Expansion of Open Banking
Open banking is another trend shaping the future of data aggregation. This practice allows third-party developers to access bank data through APIs. It promotes transparency and fosters innovation in financial services.
Considerations for Open Banking
- Consumer Empowerment: Users have greater control over their financial data, enabling them to make informed decisions.
- Increased Competition: As new players enter the market, traditional banks are pushed to enhance their offerings and customer service.
- Data Collaboration: Open banking facilitates collaboration among financial institutions, creating new opportunities for data sharing and aggregation.
In summary, the future of data aggregation in financial services hinges on the integration of AI and the expansion of open banking. These trends offer numerous benefits, from improved consumer experience to enhanced data-driven decision making. As the financial landscape adapts, staying informed on these trends will be vital for success.
Applications of Data Aggregation in Financial Services
Data aggregation plays a vital role in the financial services sector. Its applications foster a better understanding of market dynamics, customer behavior, and overall business operations. These applications not only improve operational efficiencies but also enhance strategic decision-making, leading to more informed financial outcomes.
Investment Management
Investment management is one of the most prominent applications of data aggregation. In this area, it allows stakeholders to analyze vast amounts of financial data from multiple sources. Investors and asset managers can access real-time market data, historical trends, and forecasts to make informed decisions. They have insights into overall market health, sectors that are performing well, and potential investment opportunities.
- Data Sources: Investment managers often use aggregated data from exchanges, financial news platforms, and analytical tools. Data can stem from public filings, earnings reports, and social media sentiments, shaping a broader view of investments.
- Portfolio Optimization: By integrating diverse data, professionals can optimize their portfolios. They can assess the implications of market correlations, volatility, and investor behavior, fine-tuning strategies based on recent data trends.
- Risk Adjustments: An aggregated data approach enables managers to adjust their investment strategies based on up-to-date risk assessments. This way, they can respond swiftly to changes in the financial landscape, safeguarding profits and minimizing losses.
Risk Assessment
Risk assessment is another significant application of data aggregation in the financial services domain. By compiling data from various sources, institutions can evaluate and manage risks effectively.
- Comprehensive Analysis: Risk assessment requires comprehensive analysis of credit, market, operational, and liquidity risks. Aggregating this information allows financial institutions to see potential threats from multiple angles. This is critical for making essential operational adjustments and ensuring regulatory compliance.
- Predictive Modeling: Advanced analytics and machine learning can be applied to aggregated data for predictive modeling. Financial institutions utilize historical data to identify potential risks. This foresight helps them prepare for volatility, manage capital reserves, and optimize overall risk management frameworks.
- Regulatory Compliance: Regulatory bodies often demand rigorous risk assessment protocols. By employing data aggregation practices, financial services can ensure they meet these compliance requirements while maintaining transparency and accountability.
Case Studies of Successful Data Aggregation
Case studies provide real-life examples that articulate the application and effect of data aggregation in the financial services sector. They highlight how both fintech companies and traditional banks leverage aggregated data to enhance their services, improve customer experiences, and optimize decision-making. Examining these case studies not only illustrates the effectiveness of data aggregation but also reveals the challenges and adaptations required in a rapidly evolving financial landscape.
Fintech Innovations
Fintech companies are at the forefront of using data aggregation to innovate financial services. One prominent example is Plaid, which connects consumer bank accounts to various financial applications. Plaid aggregates transaction data from thousands of banks, enabling users to access their financial information seamlessly. This integration allows applications to offer personalized insights, budgeting tools, and investment recommendations. The success of this model illustrates how effective data aggregation can lead to a better user experience.
Another example is Robinhood, a trading app that provides commission-free stock trading. By aggregating data from multiple sources, Robinhood generates insights on market trends, enabling users to make informed trading decisions without the usual barriers to entry. The ease of access and intelligent insights have attracted millions of users, showing the power of data aggregation in democratizing finance.
Traditional Banks Adapting to New Technologies
Traditional banks have also recognized the need to adopt data aggregation practices to remain competitive. JPMorgan Chase, for instance, has invested heavily in technology to improve its data management capabilities. By aggregating client data across multiple platforms, the bank can offer tailored financial advice and products. This approach not only enhances customer satisfaction but also builds long-term loyalty in a crowded market.
Moreover, Wells Fargo has implemented data aggregation systems to streamline its operations and enhance decision-making. By centralizing data from various departments, Wells Fargo can generate comprehensive reports that inform strategy and operational improvements. This transition showcases how legacy financial institutions can benefit from adopting modern data aggregation technologies.
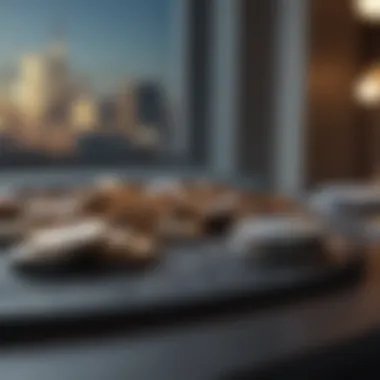
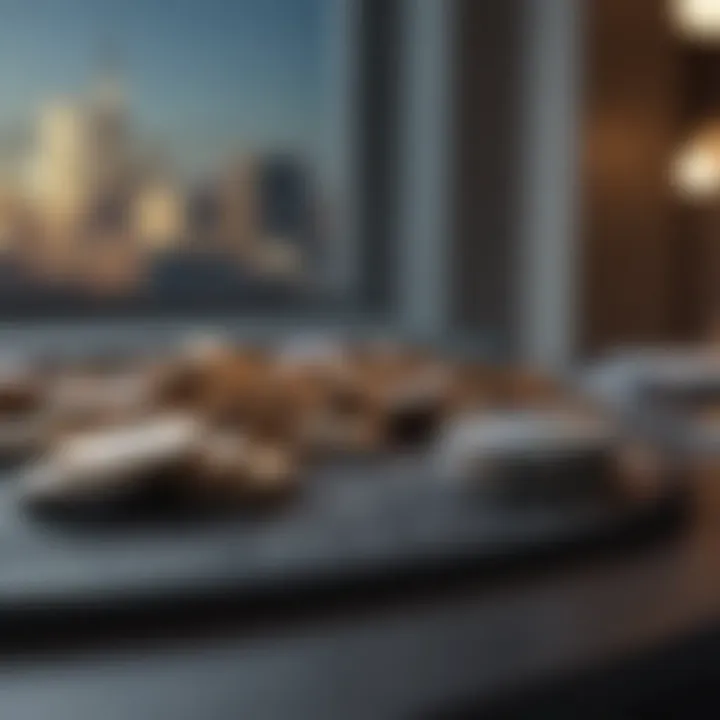
Understanding how companies use data aggregation can help investors recognize valuable trends in the financial services sector.
In summary, the exploration of case studies in data aggregation underscores its vital role in the success of both fintech innovations and traditional banks. These examples exemplify the practical advantages of utilizing aggregated data to drive growth and enhance consumer engagement.
The Role of Data Aggregation in Personal Finance
Data aggregation plays a crucial role in personal finance management. It enables individuals to have a comprehensive view of their financial situation through the collection and analysis of data from various sources. With so many financial products and services available today, it can be challenging for users to track their expenses and investments efficiently. Aggregating this data provides clarity and allows for better financial decision-making.
By utilizing data aggregation tools, individuals can consolidate information from their bank accounts, credit cards, investments, and loans into a single platform. This not only simplifies the process of monitoring finances but also aids in identifying spending patterns and opportunities for saving. Understanding one’s financial habits is essential for effective budgeting and goal setting.
However, it also requires sensitive handling of personal information. Users must consider the security measures that their chosen aggregation tools employ. A breach in data privacy can lead to significant financial repercussions. Therefore, understanding how and where data is collected can offer reassurance to consumers.
Budgeting and Expense Tracking
Budgeting is a fundamental aspect of personal finance, and data aggregation significantly enhances this process. When users aggregate their data, they gain precise insights into their income and expenditures. This information is critical for creating a realistic budget that reflects actual financial behaviors.
By analyzing consolidated data, individuals can see where they spend most of their money, identify unnecessary expenses, and allocate funds towards savings or investments. The following benefits are notable:
- Increased Awareness: With clear insights into their financial habits, users can better manage their money.
- Problem Detection: Aggregated data helps in identifying unexpected expenses or spending spikes.
- Goal Tracking: Users can set financial goals and track their progress against these objectives with aggregated insights.
Financial apps that offer budgeting and expense tracking functionalities help users visualize their financial health. This visualization often comes in the form of graphs and charts, making it easier for users to understand their financial status at a glance.
Investment Portfolio Management
Data aggregation also plays a vital role in managing investment portfolios. Investors increasingly rely on comprehensive data analysis to make informed decisions about asset allocation and diversification.
By bringing together different types of investment data—such as performance metrics, risk assessments, and market trends—aggregated insights help investors to:
- Optimize Portfolios: Users can regularly review their portfolios based on current market conditions and personal financial goals.
- Monitor Performance: Keeping track of multiple investments becomes more manageable when all data is aggregated in one place. This allows for timely decision-making.
- Risk Assessment: Understanding the risk associated with each investment becomes clearer through comprehensive data analysis.
Many modern investment platforms provide data aggregation features that allow users to see their entire portfolio's performance in real-time. This accessibility to detailed financial data enables more strategic investment strategies.
"The fundamental principle of data aggregation is that it puts the power of information into the hands of the consumer, enhancing their financial capabilities."
Data Visualization and Analysis in Financial Services
In the world of finance, making sense of vast amounts of data is essential for effective decision-making. Data visualization and analysis serve as critical tools that translate complex datasets into comprehensible visual formats. This simplification enables easier analysis and quicker insights. It is important to appreciate how these processes help financial institutions and investors alike to navigate the increasingly intricate financial landscape.
Data visualization allows stakeholders to identify patterns, trends, and anomalies in large datasets. By presenting data graphically, analysts can quickly grasp significant deviations and correlations that might not be easily visible in raw data. Moreover, effective visualization aids in communicating findings to other stakeholders, ensuring everyone is on the same page regarding performance metrics and investment strategies.
Tools for Visualization
There is a plethora of tools available for data visualization in financial services. Some of the most widely used include:
- Tableau: Known for its powerful visualization capabilities, Tableau empowers users to create interactive and shareable dashboards.
- Power BI: Developed by Microsoft, Power BI integrates seamlessly with other Microsoft products. It offers robust reporting features and data connectivity options.
- Looker: This tool focuses on data exploration, allowing users to dissect data with ease while encouraging collaboration among teams.
- D3.js: A JavaScript library that enables the creation of sophisticated interactive visuals. Although it requires programming knowledge, it offers ultimate flexibility and customization.
These tools offer a range of functionalities, catering to different organizational needs. They facilitate the elaboration of visual data narratives that can lead to more informed decisions.
Interpreting Data Insights
Interpreting data insights derived from visualizations is an art and science. Successful interpretation requires a clear understanding of the context surrounding the data. Investors and analysts must cultivate the ability to connect visuals with business outcomes, trends, and market dynamics. Here are some key considerations:
- Context: Every dataset exists within a wider context. Understanding trends in economic indicators and market conditions adds depth to the visualized data.
- Comparative analysis: By contrasting current data with historical trends, stakeholders can gauge performance and anticipate future turns in the financial market.
- Alertness to biases: It’s essential to recognize potential biases in data interpretation. A misguided reading can lead to poor investment choices or decisions that may negatively impact financial health.
- Collaboration: Engaging with colleagues to discuss insights derived from visualizations promotes diverse perspectives and enriches evaluation.
To emphasize the importance of data insights, consider this:
"Data is the new oil, but oil has to be refined to be useful."
This quote highlights the necessity for clear interpretation of data to generate actionable insights that drive success.
Impact of Data Aggregation on Consumer Behavior
In the evolving financial services landscape, data aggregation plays a critical role in shaping consumer behavior. It allows firms to collect and analyze large sets of data about customer interactions, preferences, and activities. Such insights are pivotal as they directly impact how consumers make investment decisions and their overall loyalty to financial institutions.
Influence on Investment Choices
Data aggregation has a profound influence on the investment choices consumers make. With access to comprehensive market data, customers can evaluate possible investments with greater clarity. For instance, investors can analyze historical performance metrics, market trends, and peer comparisons.
"Data-driven insights enable consumers to make informed choices rather than relying on guesses or outdated information."
By using platforms that aggregate data, consumers are empowered to compare various products, understand the risks involved, and predict future performance. They can utilize tools like portfolio trackers and investment calculators that rely on aggregated data for precision. Notably, this leads to a shift where consumers favor organizations that provide transparent, easily accessible information over those that do not. This transparency cultivates trust and encourages more active engagement in investment strategies.
Customer Loyalty and Retention
Customer loyalty is increasingly tied to how well financial services utilize aggregated data. When a firm seamlessly integrates data into its service offerings, it enhances customer experience. Personalized services and tailored recommendations arise from effective data aggregation, making clients feel valued and understood.
Businesses that harness the power of data aggregation are better equipped to address customer needs promptly. By analyzing patterns from past behaviors and preferences, these firms can craft targeted offers that resonate strongly with individual consumers. As a result, customers are more likely to remain loyal to entities that consistently deliver on their expectations through customized experiences.
Moreover, retaining customers is often cheaper than acquiring new ones. By focusing on nurturing existing relationships through data-driven approaches, institutions can build a more loyal clientele.
Epilogue
The conclusion of this article serves as a critical reflection on the multifaceted nature of data aggregation in financial services. It encapsulates the key insights discussed throughout various sections, emphasizing the significant impact of data-driven decision-making. As financial markets become increasingly complex, the ability to aggregate and analyze data effectively is essential for both investors and financial institutions.
Summary of Key Points
A few pivotal points emerge from our examination:
- Enhanced Decision-Making: Data aggregation fosters better decision-making by consolidating information from multiple sources. This leads to more informed strategies in investment management and risk assessment.
- Regulatory Awareness: Financial professionals must understand the regulatory environment influencing data practices. Compliance is not merely a legal obligation but also an operational necessity that can refine practices and build trust with clients.
- Technological Integration: The incorporation of advanced technologies such as machine learning and cloud computing has revolutionized the data aggregation processes. These innovations enhance speed and accuracy, enabling firms to respond swiftly to market changes.
- Consumer Behavior Insights: Data aggregation offers a window into consumer behavior, presenting opportunities to forge deeper connections and foster loyalty among clients. Understanding how consumers make investment choices can guide service enhancements.
- Future Trends: Anticipated trends, like the rise of open banking, signal a shift towards more collaborative financial ecosystems, which will shape how data is collected and utilized moving forward.
In summary, the significance of data aggregation cannot be overstated in the current financial landscape. Mastery of this discipline enables investors to navigate complexities, comply with changing regulations, and ultimately achieve superior financial outcomes. As technology continues to evolve, staying abreast of data aggregation methodologies and trends will be paramount for success.